InstantIR: Revolutionizing Blind Image Restoration
By Horay AI Team|
Introduction
In the realm of image processing and restoration, a groundbreaking development has emerged that promises to transform how we approach image enhancement. Instant-reference Image Restoration (InstantIR), developed by the InstantX team, introduces a novel blind image restoration (BIR) method that combines state-of-the-art restoration capabilities with an innovative previewing mechanism, setting new standards for both efficiency and user experience.
This blog will dive into the innovation behind InstantIR, how it redefines efficiency in BIR, and why it holds immense potential for applications from photography to medical imaging. We’ll also explore what sets it apart from traditional methods, making it an invaluable tool for professionals and enthusiasts alike.
Understanding Blind Image Restoration (BIR)
Definition: BIR refers to the process of recovering high-quality images from degraded observations without explicit knowledge of the degradation process.
BIR aims to "realize realistic image reconstruction on general images with general degradations". Traditional BIR approaches struggle to recover images accurately due to varying unknown degradation factors, like random noise, blur, or compression artifacts. Solutions typically require extensive computation and fail to dynamically adapt to diverse conditions in real time, making them less practical for everyday use.
InstantIR addresses these limitations, bringing rapid, intelligent restoration to images without the cumbersome need for external metadata or prior degradation analysis. This approach makes high-quality image restoration available to anyone, regardless of the input image’s original condition or specific issues, effectively broadening the scope of applications.
How InstantIR Works: A Closer Look at the Technology
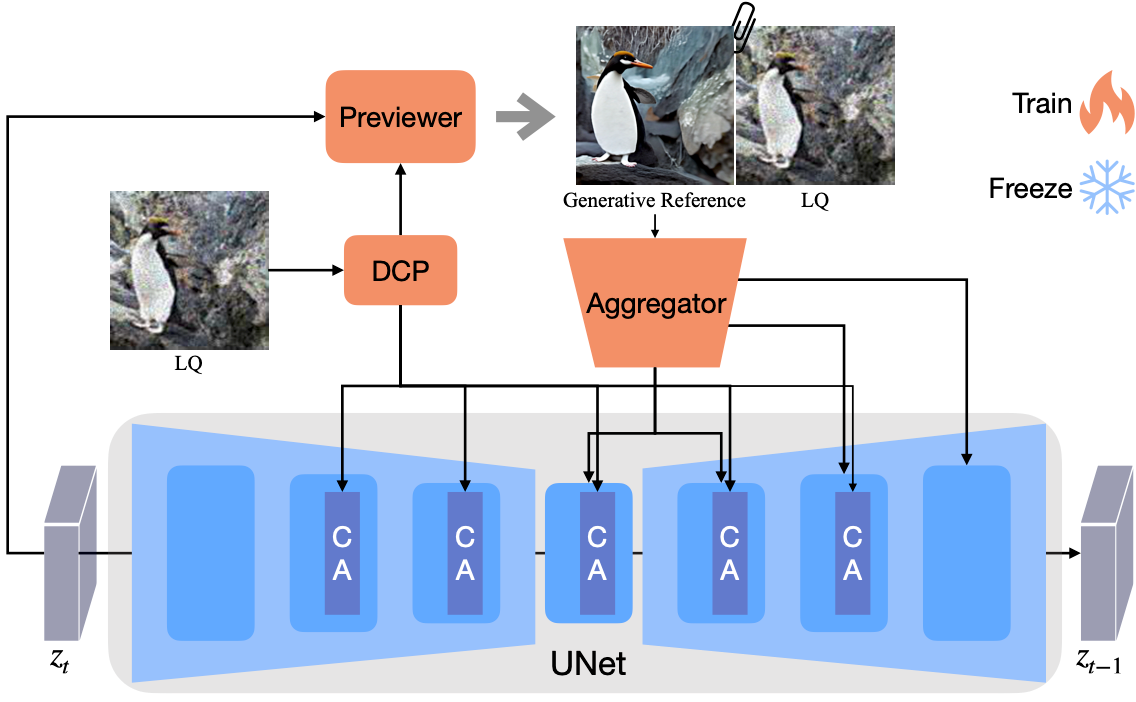
This "InstantIR pipeline" is implemented based on pre-trained SDXL model, and this diagram visually represents the process behind InstantIR’s novel BIR method.
1. DCP (Dynamic Condition Processor)
The process begins with an input image labeled "LQ" (Low Quality), which is fed into the DCP module. This module encodes a compact representation of the LQ image, capturing essential information about the image's conditions to initiate diffusion generation, without requiring detailed knowledge about the degradation itself.
2. Previewer
The encoded information from the DCP is sent to the Previewer module, where generates a restoration preview at each diffusion time-step. This component decodes the representation into a "generative prior," which is a reference image generated by the model. This generative reference acts as a high-quality version of the LQ image, providing a target or preview of what the restored image could look like.
3. Aggregator
The Aggregator module plays a central role by merging both the generative reference and the LQ image into a set of sampling conditions. Feasible details of the input image discovered from the Preview module will be further fused in the Aggregator module to preserve fidelity.
4. UNet Model
The UNet model at the bottom of the diagram receives these conditions to process the image through its architecture. UNet uses a combination of "C" and "A" that blocks to apply the generative and encoded conditions to different parts of the image, enhancing it iteratively.
5. Result
The output of the UNet model is a high-quality restored image, created by iteratively refining the input LQ image based on the generative prior and other aligned conditions.
Key Advantages of InstantIR over Traditional BIR Methods
- Adaptive Processing for Diverse ConditionsTraditional BIR techniques often struggle when degradation types vary widely. InstantIR’s adaptive processing adjusts itself based on detected degradation types, offering a customized restoration process for each image. Whether dealing with blur, noise, or pixelation, InstantIR can always provide specialized handling, which leads to superior results across a wide range of image issues.
- Speed and Efficiency with Real-Time FeedbackMany image restoration models suffer from long processing times, especially when addressing complex degradations. InstantIR’s previewing mechanism provides immediate feedback, allowing users to experiment with parameters in real-time and make adjustments without long waits. This efficiency is a game-changer for users needing quick results without sacrificing quality.
- Enhanced Quality with Fine Detail RecoveryIn traditional BIR methods, recovering fine details can be challenging, particularly when dealing with high levels of noise or compression artifacts. InstantIR has incorporated advanced algorithms for texture recovery, enabling it to restore intricate details even in heavily degraded images. This makes it ideal for fields like digital art, historical preservation, and any application where detail preservation is critical.
What's Next for InstantIR
The InstantX team has already looked ahead to enhance InstantIR further. What they mentioned in their essay of INSTANTIR: BLIND IMAGE RESTORATION WITH INSTANT GENERATIVE REFERENCE, is that "Future work will explore approach to improve the interaction between generative prior and conditions, as well as ways to refine the previewer to produce more reliable references".
Therefore, the interaction between the generative prior and condition encodings would hopefully be improved. By refining how these elements work together, the team hopes to create even more effective restoration conditions, allowing for smoother and more precise image restoration. Additionally, they plan to improve the previewer module to produce more accurate generative references. A more refined previewer would better guide the restoration process, ensuring that the generated references are closer to the intended high-quality outcome. These advancements could make InstantIR not only faster and more versatile but also more consistent and reliable in delivering top-notch image restoration results.
Conclusion: Redefining Image Restoration with InstantIR
InstantIR brings a fresh approach to BIR with its dynamic previewing mechanism and high-quality output. By addressing both speed and customization, it makes BIR accessible and efficient for diverse applications. Whether you’re a photographer, scientist, or everyday user, InstantIR offers the tools needed to bring clarity, detail, and life back into degraded images.
With InstantIR, InstantX has set a new standard in the field, paving the way for smarter, faster, and more intuitive image restoration solutions.