Introducing QwQ 32B-preview: A Breakthrough in Reasoning AI
By Horay AI Team|
Introduction
Note: This is the pronunciation of QwQ: /kwju:/ , similar to the word “quill”.
In the rapidly evolving landscape of artificial intelligence, reasoning models have emerged as a fascinating frontier of technological innovation. These AI systems aim to simulate human-like reasoning capabilities, pushing the boundaries of problem-solving and decision-making. At the forefront of this exciting development, the Qwen Team from Alibaba Cloud has unveiled QwQ (Qwen with Questions) - an open-source experimental research model that promises to revolutionize AI reasoning and analytical capabilities.
Model Specifications
The QwQ-32B-Preview is a sophisticated AI model with impressive technical specifications that set it apart in the current AI ecosystem. Built on a transformer architecture, the model incorporates advanced technologies including RoPE (Rotary Position Embedding), SwiGLU activation, RMS Normalization, and Attention QKV bias. With a substantial 32.5 billion parameters (31.0 billion non-embedding), the model then comprises 64 layers and utilizes a sophisticated attention mechanism with 40 heads for Q and 8 heads for KV.
One of the most remarkable features of QwQ is its extensive context length, supporting a full 32,768 tokens. This substantial context window allows the model to maintain coherence and depth in complex reasoning tasks, enabling more nuanced and comprehensive responses compared to many existing models.
Impressive Performance Metrics
The QwQ model has demonstrated exceptional performance across various benchmarks, positioning itself as a competitive reasoning AI with capabilities that rival prominent models like OpenAI's offerings. Its performance metrics are particularly noteworthy:
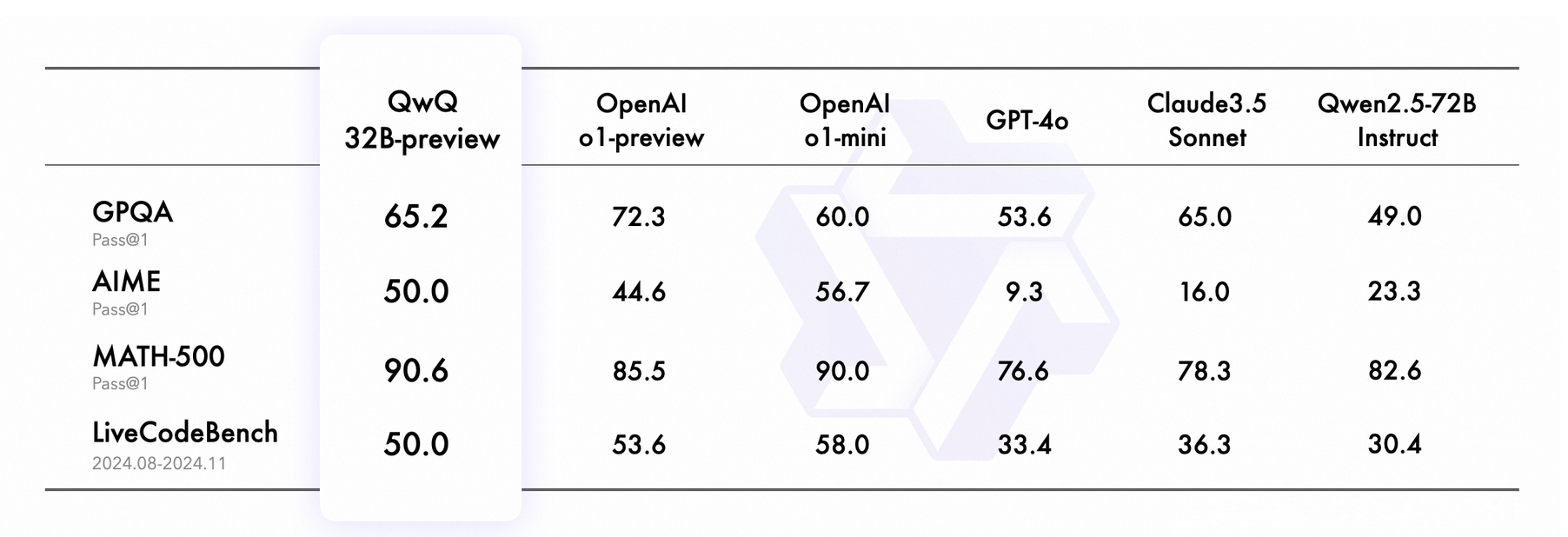
The performance of QwQ 32B-preview across different benchmarks highlights its versatility and strength in various domains. On the Graduate-level Question Answering (GPQA) benchmark, QwQ achieved an impressive score of 65.2%. While this result is competitive with Claude3.5 Sonnet (65.0%), it falls short of OpenAI o1-preview's leading performance at 72.3%. Nevertheless, QwQ's achievement underscores its advanced capabilities in scientific reasoning, making it a reliable tool for addressing complex questions in this domain.
In mathematical problem-solving, QwQ truly scored 50.0% on the AIME benchmark, showcasing its balanced approach to math-focused reasoning. However, its performance on MATH-500 was extraordinary, reaching an exceptional 90.6%. This score positions QwQ ahead of other models such as GPT-4o, demonstrating its mastery in solving advanced mathematical problems across diverse topics.
QwQ also delivers robust results in programming tasks, scoring 50.0% on the LiveCodeBench benchmark. This metric reflects its ability to handle real-world coding scenarios effectively. Its consistent ability to interpret and solve coding problems highlights its potential as a versatile programming assistant.
Overall, QwQ's performance reveals a well-rounded model with standout capabilities in mathematics and scientific reasoning. Its proficiency in answering complex queries, such as the challenging "Strawberry Question," further demonstrates its precision and adaptability. Although there is room for improvement in certain areas like GPQA and AIME, QwQ remains a strong contender in the competitive landscape of AI models, offering a broad range of applications and practical uses.
Advanced Reasoning Capabilities
What truly distinguishes QwQ is its sophisticated reasoning methodology. The model doesn't simply provide answers but engages in a complex reasoning process. It demonstrates the ability to perform multi-step reasoning, constructing intricate thought processes that involve deep introspection. This includes:
- Questioning its own assumptions
- Participating in thoughtful self-dialogue
- Analyzing each step of its reasoning process
This meta-cognitive approach allows QwQ model to generate more nuanced and reflective responses, mimicking human-like reasoning more closely than traditional language models.
Insights from the Community
This recent YouTube video titled "Yup, QwQ is CRACKED: Prompt Chaining with Qwen and QwQ reasoning model (Ollama + LLM)" provides fascinating real-world insights into QwQ's capabilities and potential applications. The video explores both the strengths and limitations of the QwQ model, offering a practical perspective on its implementation.
1. Prompt Chaining: A Game-Changing Technique
One of the most exciting developments discussed in the video is the concept of prompt chaining. This innovative technique involves using the output of one prompt as the input for another, effectively creating a more sophisticated and nuanced AI interaction. The Youtuber demonstrated this method using the Ollama Qwen 2.5 coder model, showcasing how sequential prompting can significantly enhance AI performance.
2. Practical Applications
The video also highlights several practical applications of prompt chaining, with a particular focus on content generation. For instance, the speaker illustrated a two-step process for generating SEO-optimized titles. This approach not only improves output quality but also demonstrates the model's ability to engage in complex, multi-step reasoning tasks:
- The first prompt could serve as a reasoning engine, generating potential titles
- The second prompt will extract and refine these titles using a lighter model
This video concludes with an optimistic outlook on local AI models, suggesting that solutions like QwQ represent a promising path forward in AI development. The speaker hints at future content, including predictions for 2025, and encourages community engagement and continued exploration of prompt engineering techniques.
Limitations and Considerations
Despite its impressive capabilities, QwQ is in fact still an experimental preview release with several important limitations that users and researchers should be aware of:
Language Mixing remains a challenge, with the model occasionally switching between languages unexpectedly, which can affect response clarity. There's also a tendency to enter Recursive Reasoning Loops, potentially generating lengthy responses without reaching a conclusive answer.
Safety and Ethical Considerations are paramount across the fields, especially for the AI world. The model requires enhanced safety measures to ensure reliable and secure performance. Users are advised to exercise caution during deployment and carefully evaluate its outputs. At the same time, while QwQ excels in mathematical and coding domains, it still has some room for improvement in benchmarks like common-sense reasoning and nuanced language understanding.
Accessing QwQ
Researchers and developers interested in exploring this groundbreaking model can access it through multiple platforms:
- GitHub: https://github.com/QwenLM/Qwen2.5
- HuggingFace Model: https://huggingface.co/Qwen/QwQ-32B-Preview
- ModelScope Model: https://modelscope.cn/models/Qwen/QwQ-32B-Preview
- HuggingFace Demo: https://huggingface.co/spaces/Qwen/QwQ-32B-preview
Conclusion
The Qwen Team's reflective conclusion captures the spirit of this innovative endeavor: "We don't know precisely where this journey leads, but we continue forward with unwavering determination - toward truth, toward intelligence, toward the realm where amazing happens." As AI continues to evolve, models like QwQ-32B-preview represent significant steps toward more intelligent, reflective, and nuanced artificial reasoning systems. While not perfect, they offer a glimpse into a future where AI can engage in more human-like cognitive processes.
Last but not the least, stay tuned for more updates on this exciting technological frontier!