Mastering Creativity with Precision: How ControlNet Transforms Text-to-Image AI
By Horay AI Team|
Introduction
The world of AI-generated imagery is evolving rapidly, with new models and techniques pushing the boundaries of creativity and precision. Among these innovations, ControlNet has emerged as a groundbreaking tool, enabling greater control over the creative process in text-to-image generation. This innovative neural network technique has offered unprecedented control and precision in creating visual content, continuing to bridge the gap between textual descriptions and highly customized imagery.
What is ControlNet?
ControlNet is a neural network model designed to refine and guide the creative process of text-to-image AI systems, such as Stable Diffusion. It represents a significant advancement in generative AI, providing users with fine-grained control over image generation processes. Unlike traditional text-to-image models that produce images based solely on textual prompts, ControlNet now allows for sophisticated manipulation of visual elements through additional input conditions.
Technical Architecture and Functionality
The core innovation of ControlNet lies in its ability to maintain the creative power of diffusion models while introducing precise spatial control. By incorporating additional conditioning inputs, such as edge maps, depth maps, segmentation masks, or pose estimations, the technology enables users to guide the image generation process with remarkable accuracy.
At its core, ControlNet utilizes a unique neural network architecture that works alongside existing diffusion models like Stable Diffusion. The system operates by:
- 1. Accepting multiple types of conditional inputs alongside text prompts
- 2. Maintaining the original model's generation capabilities
- 3. Introducing fine-grained control mechanisms without extensive retraining
For those who are seeking a practical understanding of ControlNet's implementation, the video tutorial "ControlNet Stable Diffusion Tutorial In 8 Minutes" provides a comprehensive guide on using ControlNet, an extension that enhances image generation control by applying various reference images to create control maps like pose and depth. Designed for those interested in exploring ControlNet within the Stable Diffusion framework, this concise tutorial provides a comprehensive overview of installation procedures on Automatic1111 version 1.6, including downloading necessary models from Hugging Face, and some related user interface fundamentals for activating control units and manipulating reference images. This video serves as an excellent starting point for both designers, and AI researchers looking to gain practical insights into how ControlNet functions within a specific text-to-image model.
Key Features of ControlNet
- Flexible Inputs
- Accepts a variety of input formats: edge maps, depth maps, segmentation maps, human poses, and more.
- Supports partial inputs, meaning users can provide minimal guidance and still see impactful results.
- Preservation of Artistic Freedom
- While ControlNet follows the provided inputs, it leaves room for the AI's inherent creativity, blending user intentions with the model’s interpretive capabilities.
- Improved Consistency
- Addresses the inconsistency often seen in text-to-image models by adhering more closely to user-provided data, making it invaluable for precise designs.
- Compatibility
- Works with popular diffusion models like Stable Diffusion and can be easily integrated into existing workflows.
Practical Demonstrations of ControlNet Capabilities
Edge Detection Control
Edge detection represents a fundamental method of guiding image generation with structural precision. Through this approach, ControlNet uses a detailed edge map as a conditional input, allowing users to define the precise structural outline of an image before generation begins.
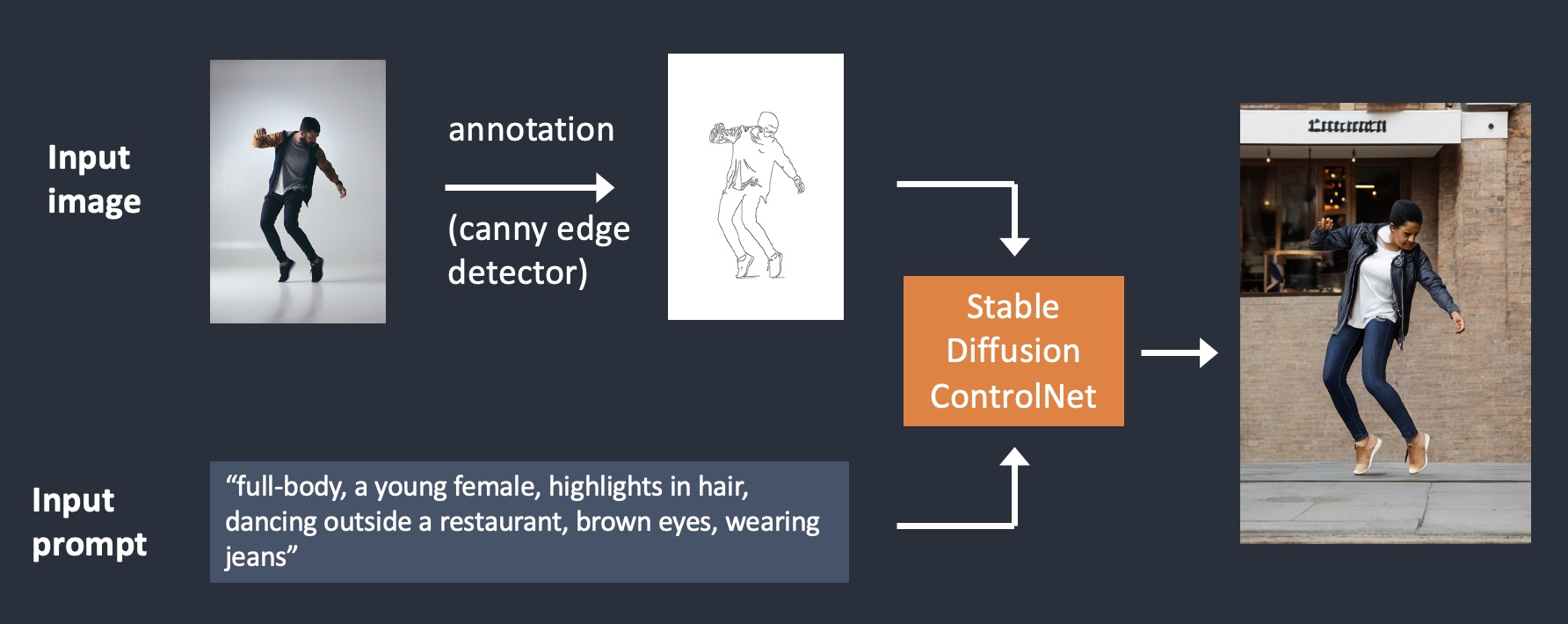
This image illustrates the power of ControlNet with Canny edge conditioning in Stable Diffusion. The process begins with an input image, which could be a photograph or any visual reference. This image will serve as the foundation for creating a structurally accurate output. The next step involves edge detection, achieved through a technique like the Canny Edge Detector. This method extracts the key structural outlines from the input image, focusing on defining the subject's contours and pose. The resulting edge map is a simplified line-based version of the original image, which provides crucial guidance for the generation process.
Alongside the edge map, the user provides a text prompt that specifies additional details, such as the subject’s appearance, environment, and overall styling. Textual inputs could always add some contextual and stylistic layers to the structured guidance provided by the edge map. These inputs are then processed by Stable Diffusion integrated with ControlNet. ControlNet ensures that the generated image remains faithful to the edge map’s structure while incorporating the prompt’s creative details. The result will be a highly realistic output that preserves the original pose and proportions but transforms the context and style to match the user’s description.
Human Pose Detection and Generation
Human pose detection offers an even more nuanced form of image control. ControlNet can interpret and replicate complex human poses with remarkable accuracy, making it invaluable for fields ranging from animation to fashion design.
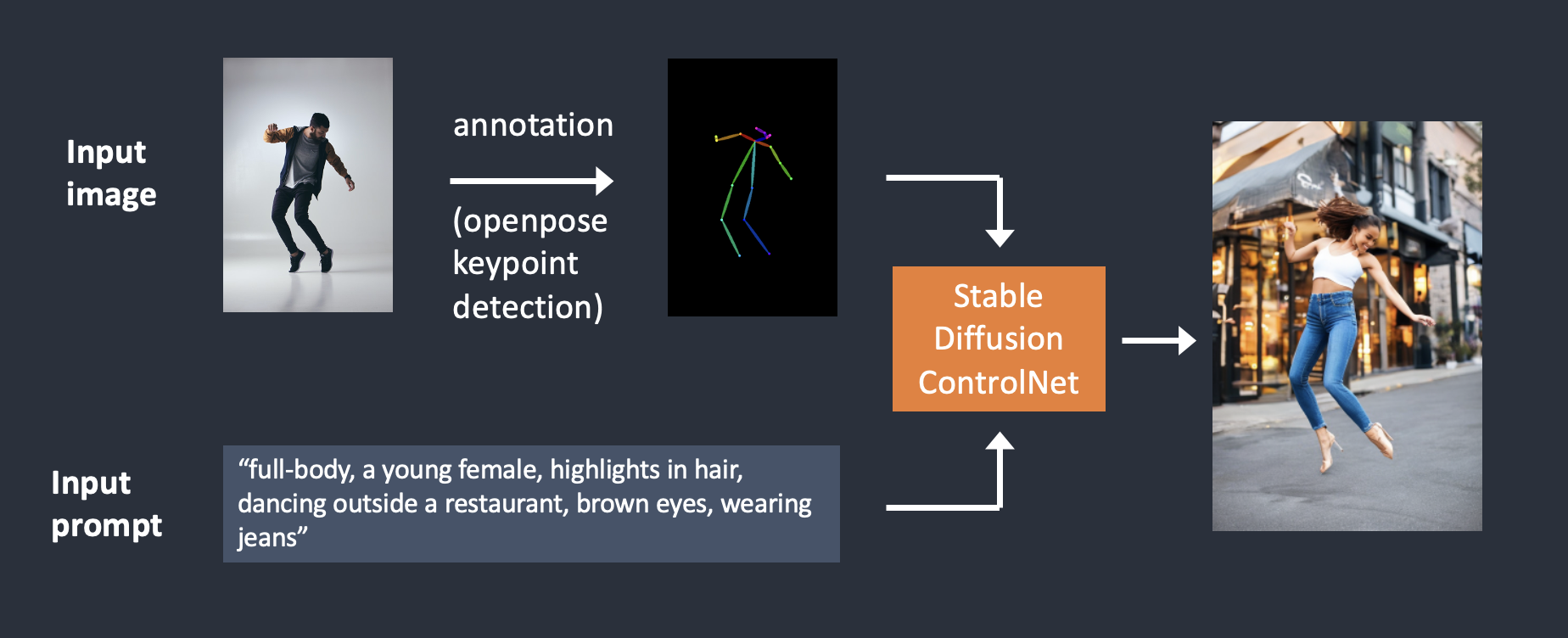
This image showcases the ControlNet workflow using OpenPose, a groundbreaking method for blending structural precision with creative freedom in AI image generation. It still begins with an input image, which serves as a visual reference. The next step utilizes OpenPose keypoint detection, which analyzes the input image to extract keypoints representing critical body positions. These keypoints correspond to parts such as the head, arms, torso, and legs, forming a skeletal outline of the subject. This skeletal structure, known as the control map, encodes the subject’s pose and movement while removing unnecessary visual details. It acts as a structural guide, ensuring the generated output retains the original pose.
In parallel, a text prompt adds contextual and stylistic details to the generation process. Both the control map and text prompt are fed into Stable Diffusion integrated with ControlNet. The integration of these two inputs enables the AI to maintain a balance between structural accuracy and artistic freedom. The control map ensures the generated image adheres to the keypoints and body proportions from the input, while the text prompt determines the finer details, such as the character’s features, clothing, and background.
These above workflows all highlight the versatility of ControlNet with Stable Diffusion, making it a powerful tool for applications such as character design, animation, and recreating specific poses in art. On top of them, ControlNet can also work on Depth Map Conditioning which enables control over three-dimensional spatial relationships, or Segmentation Mask Control to provide granular control over specific image regions. By seamlessly integrating structural guidance and creative input, ControlNet always allows users to achieve precise, visually striking results.
Where to Learn More
To dive deeper into ControlNet and experiment with its capabilities, check out the following resources:
- ControlNet GitHub Repository: Explore the technical details and access open-source code.
- Runway ML: Experiment with ControlNet on this user-friendly creative platform.
- Hugging Face: Learn about ControlNet and various AI tools, and find pretrained ControlNet models.
- Stable Diffusion: Discover the ecosystem where ControlNet shines.
Conclusion
ControlNet stands at the forefront of a transformative shift in generative AI, offering unprecedented control and creativity in image generation. By providing sophisticated conditioning mechanisms, this technology empowers creators across multiple domains to bring their most intricate visual concepts to life with remarkable precision.
As AI-driven creativity becomes mainstream, tools like ControlNet will play a pivotal role in democratizing design. The combination of user input with AI’s generative capabilities ensures a balanced approach, where both precision and creativity thrive. With further development, ControlNet could integrate with other modalities, such as video generation or 3D modeling, further expanding its influence.
Stay tuned, then explore possibilities of ControlNet and unlock new levels of creativity!!!